forked from snxraven/ravenscott-blog
470 lines
18 KiB
Markdown
470 lines
18 KiB
Markdown
|
# AI Log Monitoring System
|
||
|
|
||
|
# Source
|
||
|
|
||
|
## https://git.ssh.surf/snxraven/ai-nginx-log-security
|
||
|
|
||
|
|
||
|
## Introduction
|
||
|
|
||
|
The AI Log Monitoring System is a powerful and extensible solution designed to enhance security monitoring by integrating AI-based analysis into traditional NGINX log management.
|
||
|
|
||
|
By continuously tailing log files and utilizing an AI model to analyze log entries in real-time, this system automates the detection of security threats, sends alerts to designated channels (like Discord), and manages conversation history for ongoing log analysis.
|
||
|
|
||
|
The solution is composed of two primary scripts:
|
||
|
|
||
|
- `ai_log.js`: Handles log monitoring, buffering, and sending logs to a backend for AI processing.
|
||
|
- `ai_log_backend.js`: Manages AI-based analysis, conversation history, and exposes an API for interacting with the AI service.
|
||
|
|
||
|
## Screenshots
|
||
|
|
||
|
Here are some screenshots of the application in action:
|
||
|
|
||
|
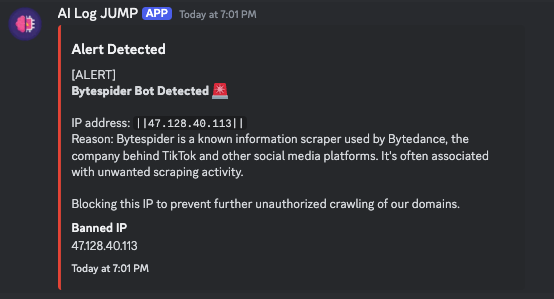
|
||
|
|
||
|
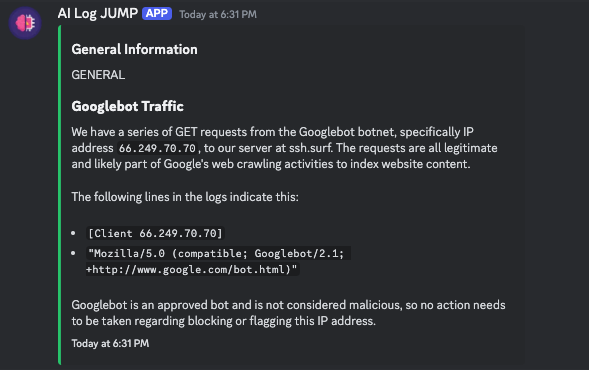
|
||
|
|
||
|
|
||
|
## Prerequisites
|
||
|
|
||
|
Before setting up and running the AI Log Monitoring System, ensure you have the following components installed and configured on your machine:
|
||
|
|
||
|
- **Node.js**: Version 14.x or higher is required to run the JavaScript code.
|
||
|
- **npm**: Version 6.x or higher is needed to manage project dependencies.
|
||
|
- **Docker**: Required for running the AI model, particularly if using a containerized llama 3.1 model for processing.
|
||
|
- **NGINX**: The web server generating logs that the system will monitor.
|
||
|
- **Discord**: A Discord webhook URL is necessary for sending security alerts.
|
||
|
- **llama-cpp-python[web]**: A docker container preferably although not required: [Read the Docs](https://llama-cpp-python.readthedocs.io/en/latest/server/)
|
||
|
|
||
|
|
||
|
Ensure you have administrative privileges on the machine to install and configure these dependencies.
|
||
|
|
||
|
## Installation
|
||
|
|
||
|
Clone the repository to your local machine:
|
||
|
|
||
|
```bash
|
||
|
git clone git@git.ssh.surf:snxraven/ai-nginx-log-security.git
|
||
|
cd ai-nginx-log-security
|
||
|
```
|
||
|
|
||
|
Install the required Node.js dependencies for both applications:
|
||
|
|
||
|
```bash
|
||
|
npm install
|
||
|
```
|
||
|
|
||
|
## Configuration
|
||
|
|
||
|
### Environment Variables
|
||
|
|
||
|
Environment variables play a critical role in configuring the behavior of the AI Log Monitoring System.
|
||
|
|
||
|
They allow you to customize log directories, adjust token limits, and set up necessary credentials.
|
||
|
|
||
|
Create a `.env` file in the root of your project and populate it with the following variables:
|
||
|
|
||
|
```bash
|
||
|
# General Configuration
|
||
|
DEBUG=true # Enable detailed logging output for debugging
|
||
|
WEBHOOKURL=https://discord.com/api/webhooks/your-webhook-id # Discord webhook URL for sending alerts
|
||
|
MAX_CONTENT_LENGTH=2000 # Maximum length for content scraped from web pages
|
||
|
|
||
|
# AI Service Configuration
|
||
|
TIMEOUT_DURATION=100000 # Maximum duration (in ms) for API requests to complete
|
||
|
MAX_TOKENS=8000 # Maximum tokens allowed in a conversation before trimming
|
||
|
TOLERANCE=100 # Extra tokens allowed before forcing a trim of conversation history
|
||
|
```
|
||
|
|
||
|
**Explanation of Environment Variables**:
|
||
|
|
||
|
- **DEBUG**: Enables verbose logging, including debug-level messages. Useful during development and troubleshooting.
|
||
|
- **WEBHOOKURL**: The Discord webhook URL where alerts will be sent. This should be a valid and secure URL.
|
||
|
- **MAX_CONTENT_LENGTH**: Limits the length of content extracted from web pages to avoid overloading the system or sending excessively long messages.
|
||
|
- **TIMEOUT_DURATION**: Sets a timeout for requests to the AI service, preventing the system from hanging indefinitely.
|
||
|
- **MAX_TOKENS**: Controls the total number of tokens (words + punctuation) allowed in a conversation with the AI. This prevents the AI from consuming too much memory or processing power.
|
||
|
- **TOLERANCE**: A buffer to avoid hitting the MAX_TOKENS limit exactly, ensuring smoother operation by providing a cushion before trimming conversation history.
|
||
|
|
||
|
### Directory Structure
|
||
|
|
||
|
The system monitors log files stored in a specific directory. Ensure this directory exists and is correctly set in the script:
|
||
|
|
||
|
```bash
|
||
|
mkdir -p /dockerData/logs
|
||
|
```
|
||
|
|
||
|
If your NGINX logs are stored in a different directory, update the `LOG_DIRECTORY` constant in `ai_log.js` accordingly:
|
||
|
|
||
|
```javascript
|
||
|
const LOG_DIRECTORY = '/your/custom/path/to/logs';
|
||
|
```
|
||
|
|
||
|
### Ignored IPs and Subnets
|
||
|
|
||
|
The system allows you to specify IP addresses and subnets that should be ignored during log monitoring.
|
||
|
|
||
|
This is particularly useful for filtering out trusted sources or known harmless traffic (e.g., public DNS servers).
|
||
|
|
||
|
- **Ignored IPs**: Directly listed IP addresses that should be skipped during processing.
|
||
|
- **Ignored Subnets**: Subnets specified in CIDR notation that represent ranges of IP addresses to be ignored.
|
||
|
|
||
|
To customize the ignored IPs and subnets, modify the `ignoredIPs` and `ignoredSubnets` arrays in `ai_log.js`:
|
||
|
|
||
|
```javascript
|
||
|
const ignoredIPs = ['1.1.1.1', '1.0.0.1', '8.8.8.8', '8.8.4.4'];
|
||
|
const ignoredSubnets = [
|
||
|
'173.245.48.0/20', '103.21.244.0/22', '103.22.200.0/22',
|
||
|
// Add more subnets as needed
|
||
|
];
|
||
|
```
|
||
|
|
||
|
This ensures that traffic from these sources is not flagged or acted upon, reducing false positives and focusing the AI on more critical threats.
|
||
|
|
||
|
## Usage
|
||
|
|
||
|
### Running `ai_log.js`
|
||
|
|
||
|
The `ai_log.js` script is responsible for continuously monitoring NGINX logs, buffering log entries, and sending them to the backend for analysis.
|
||
|
|
||
|
It also handles real-time actions, such as banning IP addresses and sending alerts.
|
||
|
|
||
|
To start the log monitoring process, execute the following command:
|
||
|
|
||
|
```bash
|
||
|
node ai_log.js
|
||
|
```
|
||
|
|
||
|
The script will immediately begin reading logs from the specified directory and processing them according to the rules defined in the script.
|
||
|
|
||
|
The logs will be buffered and periodically sent to the backend for AI-based analysis.
|
||
|
|
||
|
### Running `ai_log_backend.js`
|
||
|
|
||
|
The `ai_log_backend.js` script sets up an Express server that interfaces with the AI model to analyze log data.
|
||
|
|
||
|
It also manages conversation history and provides endpoints for interacting with the system.
|
||
|
|
||
|
To start the backend server:
|
||
|
|
||
|
```bash
|
||
|
node ai_log_backend.js
|
||
|
```
|
||
|
|
||
|
By default, the server will be running on `http://localhost:3001`.
|
||
|
|
||
|
This server handles incoming log data, processes it with the AI model, and returns actionable insights, including potential security alerts.
|
||
|
|
||
|
## How It Works
|
||
|
|
||
|
### Log Monitoring and Buffering
|
||
|
|
||
|
The `ai_log.js` script uses the `Tail` module to monitor NGINX log files in real-time.
|
||
|
|
||
|
As new lines are added to the logs, the script reads and buffers them.
|
||
|
|
||
|
The buffer size is configurable, allowing the system to batch-process logs before sending them to the backend.
|
||
|
|
||
|
**Key Features**:
|
||
|
|
||
|
- **Real-Time Monitoring**: Continuously monitors specified log files for new entries.
|
||
|
- **Buffering**: Collects log entries in a buffer to reduce the frequency of network requests to the backend.
|
||
|
- **Ignored Entries**: Filters out log entries from specified IPs and subnets, as well as entries matching certain patterns.
|
||
|
|
||
|
### Sending Logs to Backend
|
||
|
|
||
|
When the log buffer reaches a predefined size or a set time interval elapses, the buffered logs are sent to the backend for AI processing.
|
||
|
|
||
|
The backend analyzes the logs to detect potential security threats, generate alerts, and manage conversation history.
|
||
|
|
||
|
**Process Overview**:
|
||
|
|
||
|
1. **Buffer Accumulation**: Logs are collected in a buffer until a threshold is met.
|
||
|
2. **Buffer Flush**: The buffer is sent to the backend in one request, optimizing network usage.
|
||
|
3. **Backend Analysis**: The AI model analyzes the logs for suspicious activity or patterns.
|
||
|
|
||
|
### AI-Based Log Analysis
|
||
|
|
||
|
The backend server (`ai_log_backend.js`) leverages an AI model (e.g., llama 3.1) to analyze the logs and detect potential security threats.
|
||
|
|
||
|
The AI operates based on a custom prompt that instructs it on how to interpret the logs, which IPs to ignore, and what actions to take.
|
||
|
|
||
|
**AI Model Usage**:
|
||
|
|
||
|
- **Custom Prompt**: The AI is guided by a detailed prompt that defines its behavior and decision-making process.
|
||
|
- **Log Parsing**: The AI processes log lines to identify malicious patterns, potential attacks, or other security concerns.
|
||
|
- **Actionable Insights**: Based on the analysis, the AI generates alerts, suggests actions (like banning IPs), or provides general observations.
|
||
|
|
||
|
### Token Management and Conversation History
|
||
|
|
||
|
To ensure efficient operation and prevent resource exhaustion, the system carefully manages the number of tokens used in AI conversations.
|
||
|
|
||
|
Token management involves trimming older parts of the conversation history to stay within predefined limits.
|
||
|
|
||
|
**Token Management Strategies**:
|
||
|
|
||
|
- **Counting Tokens**: The system counts tokens for each message in the conversation history.
|
||
|
- **Trimming History**: If the token count exceeds the maximum allowed, the oldest messages are removed.
|
||
|
- **Tolerance Buffer**: A small buffer is maintained to avoid hitting the exact token limit, ensuring smoother performance.
|
||
|
|
||
|
### Security Alert Handling
|
||
|
|
||
|
When the AI detects a potential security threat, it generates an alert.
|
||
|
|
||
|
These alerts are processed by the backend and can trigger actions like banning an IP address or sending a notification to a Discord channel.
|
||
|
|
||
|
**Alert Workflow**:
|
||
|
|
||
|
1. **Detection**: The AI identifies a suspicious activity or pattern in the logs.
|
||
|
2. **Alert Generation**: The AI creates an alert message, formatted for clarity and readability.
|
||
|
3. **IP Banning**: If an IP is identified as malicious, the system can execute a ban command to block it.
|
||
|
4. **Discord Notification**: Alerts are sent to a designated Discord channel for real-time monitoring and action.
|
||
|
|
||
|
### Discord Integration
|
||
|
|
||
|
The system integrates with Discord to send alerts and notifications.
|
||
|
|
||
|
This is particularly useful for real-time monitoring, allowing administrators to receive and act on security alerts instantly.
|
||
|
|
||
|
**Integration Details**:
|
||
|
|
||
|
- **Webhook-Based Alerts**: The system uses a Discord webhook to send alerts as embedded messages.
|
||
|
- **Formatted Messages**: Alerts are formatted with titles, descriptions, and timestamps to ensure they are easy to read and understand.
|
||
|
- **IP Banning Alerts**: When an IP is banned, the system includes the IP address in the Discord alert.
|
||
|
|
||
|
## API Endpoints
|
||
|
|
||
|
The backend server (`ai_log_backend.js`) exposes several API endpoints for interacting with the AI service, managing conversation history, and controlling the system.
|
||
|
|
||
|
### POST /api/v1/chat
|
||
|
|
||
|
This endpoint processes incoming NGINX logs by sending them to the AI model for analysis.
|
||
|
|
||
|
- **Request Body**:
|
||
|
- `message`: A string containing one or more NGINX log lines.
|
||
|
|
||
|
- **Response**:
|
||
|
- A JSON object with the AI's analysis and any detected alerts.
|
||
|
|
||
|
**Example Request**:
|
||
|
|
||
|
```json
|
||
|
{
|
||
|
"message": "127.0.0.1 - - [12/Mar/2024:10:12:33 +0000] \"GET /index.html HTTP/1.1\" 200 3050"
|
||
|
}
|
||
|
```
|
||
|
|
||
|
**Example Response**:
|
||
|
|
||
|
```json
|
||
|
{
|
||
|
"role": "assistant",
|
||
|
"content": "GENERAL: No suspicious activity detected. Routine request logged."
|
||
|
}
|
||
|
```
|
||
|
|
||
|
### GET /api/v1/conversation-history
|
||
|
|
||
|
This endpoint retrieves the conversation history for the IP address making the request.
|
||
|
|
||
|
It is useful for reviewing the AI's past analyses and actions.
|
||
|
|
||
|
- **Response**:
|
||
|
- A JSON array containing the conversation history.
|
||
|
|
||
|
**Example Response**:
|
||
|
|
||
|
```json
|
||
|
[
|
||
|
{ "role": "system", "content": "You are a security AI..." },
|
||
|
{ "role": "user", "content": "127.0.0.1 - - [12/Mar/2024:10:12:33 +0000] ..." },
|
||
|
{ "role": "assistant", "content": "GENERAL: No suspicious activity detected..." }
|
||
|
]
|
||
|
```
|
||
|
|
||
|
### POST /api/v1/restart-core
|
||
|
|
||
|
This endpoint restarts the core AI service running in a Docker container.
|
||
|
|
||
|
This is useful if the AI service becomes unresponsive or needs to refresh its state.
|
||
|
|
||
|
- **Response**:
|
||
|
- A JSON object with the output of the Docker restart command.
|
||
|
|
||
|
**Example Request**:
|
||
|
|
||
|
```bash
|
||
|
curl -X POST http://localhost:3001/api/v1/restart-core
|
||
|
```
|
||
|
|
||
|
**Example Response**:
|
||
|
|
||
|
```json
|
||
|
{
|
||
|
"stdout": "llama-gpu-server\n"
|
||
|
}
|
||
|
```
|
||
|
|
||
|
### POST /api/v1/reset-conversation
|
||
|
|
||
|
This endpoint resets the conversation history for the requesting IP address, effectively starting a new session with the AI.
|
||
|
|
||
|
This can be useful for clearing outdated context and beginning a fresh analysis.
|
||
|
|
||
|
- **Response**:
|
||
|
- A JSON object confirming the reset action.
|
||
|
|
||
|
**Example Request**:
|
||
|
|
||
|
```bash
|
||
|
curl -X POST http://localhost:3001/api/v1/reset-conversation
|
||
|
```
|
||
|
|
||
|
**Example Response**:
|
||
|
|
||
|
```json
|
||
|
{
|
||
|
"message": "Conversation history reset for IP: 127.0.0.1"
|
||
|
}
|
||
|
```
|
||
|
|
||
|
## Logging
|
||
|
|
||
|
Logging is a critical component of the AI Log Monitoring System, providing insights into system operations, debugging information, and records of security actions.
|
||
|
|
||
|
### Log Levels
|
||
|
|
||
|
The system categorizes logs into different levels to help you quickly identify the nature and severity of messages:
|
||
|
|
||
|
- **INFO**: General information about the system's operations.
|
||
|
- **WARN**: Indications of potential issues that may require attention.
|
||
|
- **ERROR**: Logs generated when an error occurs during processing.
|
||
|
- **SUCCESS**: Messages that indicate successful operations or actions.
|
||
|
- **DEBUG**: Detailed messages intended for debugging purposes, enabled when `DEBUG=true`.
|
||
|
|
||
|
### Log Structure
|
||
|
|
||
|
Each log message includes a timestamp, log level, and message content. For example:
|
||
|
|
||
|
```plaintext
|
||
|
2024-03-12 10:12:33 [INFO] Starting to read log from: /dockerData/logs/access.log
|
||
|
2024-03-12 10:12:35 [DEBUG] Read line: 127.0.0.1 - - [12/Mar/2024:10:12:33 +0000] "GET /index.html HTTP/1.1" 200 3050
|
||
|
2024-03-12 10:12:35 [SUCCESS] Log buffer sent to backend successfully.
|
||
|
```
|
||
|
|
||
|
### Debugging
|
||
|
|
||
|
When `DEBUG=true`, the system provides detailed logs that include every step of the processing workflow.
|
||
|
|
||
|
This includes reading log lines, checking for ignored IPs, sending data to the backend, and receiving responses from the AI.
|
||
|
|
||
|
These logs are invaluable during development and troubleshooting, as they offer full visibility into the system's inner workings.
|
||
|
|
||
|
## Security Considerations
|
||
|
|
||
|
Security is a paramount concern when monitoring logs and responding to potential threats.
|
||
|
|
||
|
The AI Log Monitoring System includes several mechanisms to enhance security and minimize false positives.
|
||
|
|
||
|
### IP Whitelisting
|
||
|
|
||
|
The system allows you to specify IP addresses and subnets that should be ignored during analysis.
|
||
|
|
||
|
This is particularly useful for avoiding alerts from known and trusted sources, such as public DNS servers or internal IP ranges.
|
||
|
|
||
|
### Rate Limiting and Banning
|
||
|
|
||
|
To protect your infrastructure from repeated attacks, the system can automatically ban IP addresses identified as malicious.
|
||
|
|
||
|
The banning process is executed via shell commands, and the system includes a delay mechanism to prevent overloading the network with too many ban requests in a short period.
|
||
|
|
||
|
### Data Privacy
|
||
|
|
||
|
All sensitive data, such as IP addresses and conversation history, is handled securely.
|
||
|
|
||
|
The system ensures that only necessary data is stored and processed, with an emphasis on minimizing exposure to potential vulnerabilities.
|
||
|
|
||
|
## Performance Optimization
|
||
|
|
||
|
The AI Log Monitoring System is designed to be efficient and scalable, handling large volumes of log data with minimal overhead.
|
||
|
|
||
|
However, some optimizations can further enhance performance, especially in high-traffic environments.
|
||
|
|
||
|
### Managing Token Limits
|
||
|
|
||
|
By carefully managing the number of tokens in the AI's conversation history, the system prevents memory overuse and ensures faster response times. The `MAX_TOKENS` and `TOLERANCE` variables allow you to fine-tune this behavior.
|
||
|
|
||
|
### Efficient Log Parsing
|
||
|
|
||
|
The system uses regular expressions and filtering techniques to parse and analyze log files efficiently. By focusing only on relevant log entries and ignoring unnecessary ones, the system reduces processing time and improves accuracy.
|
||
|
|
||
|
## Troubleshooting
|
||
|
|
||
|
If you encounter issues while using the AI Log Monitoring System, this section provides guidance on common problems and how to resolve them.
|
||
|
|
||
|
### Common Issues
|
||
|
|
||
|
- **No Logs Detected**: Ensure that the log directory is correctly specified and that log files are present.
|
||
|
- **AI Service Unresponsive**: Restart the AI service using the `/api/v1/restart-core` endpoint.
|
||
|
- **Excessive False Positives**: Review the ignored IPs and subnets to ensure that known safe traffic is excluded.
|
||
|
|
||
|
### Restarting Services
|
||
|
|
||
|
If the AI service becomes unresponsive or you need to apply changes, use the `/api/v1/restart-core` endpoint to restart the core AI Docker container.
|
||
|
|
||
|
This refreshes the model and clears any stale states.
|
||
|
|
||
|
### Log Analysis
|
||
|
|
||
|
Review the logs generated by the system to identify potential issues. Focus on `ERROR` and `WARN` level messages to spot critical problems quickly. Use the `DEBUG` logs for deeper investigation during development or when troubleshooting specific issues.
|
||
|
|
||
|
## Customization
|
||
|
|
||
|
The AI Log Monitoring System is highly customizable, allowing you to tailor its behavior to your specific needs.
|
||
|
|
||
|
### Modifying the AI Prompt
|
||
|
|
||
|
The AI's behavior is guided by a custom prompt that defines how it should interpret log data and what actions it should take.
|
||
|
|
||
|
You can modify this prompt in `ai_log_backend.js` to adjust the AI's focus or add new rules.
|
||
|
|
||
|
**Example Customization**:
|
||
|
|
||
|
```javascript
|
||
|
const prompt = `
|
||
|
You are a security AI responsible for analyzing web traffic...
|
||
|
- Ignore any IPs within the range 10.0.0.0/8.
|
||
|
- Flag any requests to /admin as potential threats.
|
||
|
- Use emojis to convey the severity of alerts.
|
||
|
`;
|
||
|
```
|
||
|
|
||
|
### Adjusting Buffer Limits
|
||
|
|
||
|
The log buffer size determines how many log lines are collected before they are sent to the backend.
|
||
|
|
||
|
Adjust this size to balance network usage and processing frequency.
|
||
|
|
||
|
**Example Customization**:
|
||
|
|
||
|
```javascript
|
||
|
const LOG_BUFFER_LIMIT = 30; // Increase the buffer size to 30 lines
|
||
|
```
|
||
|
|
||
|
### Extending Log Parsing Capabilities
|
||
|
|
||
|
You can extend the log parsing functionality by adding new regular expressions or parsing logic to handle different log formats or detect new types of threats.
|
||
|
|
||
|
**Example Customization**:
|
||
|
|
||
|
```javascript
|
||
|
tail.on('line', async (line) => {
|
||
|
// Add custom logic to detect SQL injection attempts
|
||
|
if (/SELECT.*FROM/i.test(line)) {
|
||
|
log.warn(`Potential SQL injection detected: ${line}`);
|
||
|
}
|
||
|
});
|
||
|
```
|
||
|
|
||
|
# My Findings
|
||
|
|
||
|
The AI Log Monitoring System is an advanced and highly customizable solution for real-time security monitoring of NGINX logs. By leveraging AI-based analysis, it significantly improves the detection of potential security threats, streamlines alert notifications, and provides administrators with actionable insights through Discord integration. With features like log buffering, IP whitelisting, and token management, the system efficiently handles large volumes of log data while minimizing false positives. Its modular design, supported by two core scripts, ensures scalability and adaptability, making it a robust tool for securing web infrastructure.
|